Blog 58: Product analytics for better decision making.
- Idea2Product2Business Team
- Jun 19, 2024
- 2 min read
Updated: Aug 16, 2024
Product analytics is understanding how users engage with our product. To do this analysis, we need to collect the right data (i.e., metrics). Hence, tracking these metrics is critical. Refer blog 34 for metrics, KPIs and OKR.
The data that we capture (around user engagement and user behaviour) is visualised or analysed to generate insights. Leading to improved decision making and product optimization. Due to tech improvements, real-time data can be captured too. Data can be both semi-structured and unstructured data. In addition, to traditional structured data.
What kind of analysis can be done with the captured data?
Descriptive analytics: Gain insight from historical data with reporting, scorecards, clustering etc. For e.g., number of daily active users. Is it on the rise or decline?
Predictive analytics: Once we understand historical data, the goal is to forecast future trends, or understand the impact of hypothetical actions. For e.g., Forecast the increase in daily active users after a paid Google advertising campaign. Thus, it involves predictive modelling using statistical and machine learning techniques.
Prescriptive analytics: It enables creating systems that recommend decisions using optimization, simulation etc. It uses a combination of business rules and machine learning algorithms. For e.g., should we spend $5,000 more on Google ads to increase daily active users by 10%?
As you can see, we start with descriptive analytics and then move up the chain. In all three types, we generally try to understand influence of an independent variable (ads spend, product price, font colour, etc.) on a dependent variable (daily active users, sales, etc.).
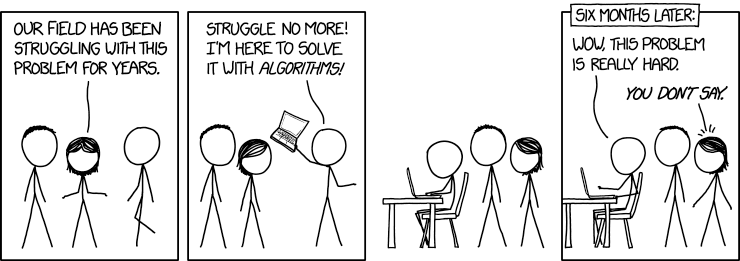
Source: An image sourced from the web
As the data and the number of independent and dependent variables increase, analysis can get overwhelming. We risk barking up the wrong tree. Exploring data without any hypothesis. Frameworks like Structured Pyramid Analysis Plan (SPAP) help us avoid this.
The Structured Pyramid Analysis Plan consists of 5 steps.
1. Formulate a SMART goal.
2. From which we deduce one or several relevant dependent variables.
3. Brainstorm to formulate specific questions regarding the above dependent variables.
4. Based on specific questions (in step 3), identify, and understand the influence of independent variables.
5. Visualisations, analysis, and charts of independent variables versus dependent variables. Generate insights.
You can read this blog on Medium – it has a detailed worked-out example.
If we are managing a well-established product with over a million active users. The amount of data generated, with its complexity, is significantly large. So is the user engagement and user behaviour data. It can be spread across diverse data sources. Retrieving and collecting the right data is crucial.
To conclude, it is advisable to be familiar with the following:
What are the different central/departmental data sources/infrastructure?
What data is available for our hypothesis?
If available? How much and at what quality?
Are there data dictionaries?
Which data will be useful?
Who understands the data?
Completeness of data (% of missing values)
Outliers/noise in data
What kind of data preparation will be required (i.e., mapping, sampling, feature extraction, selection, etc.)?
Refer to blog 83: for a list of metrics and performance tools.
Jump to blog 100 to refer to the overall product management mind map.
I wish you the best for your journey. 😊